How-to: Fine-tuning
Learn details on how to use your own private data to fine-tune a custom LLM.
Fine-tuning LLM is the process of improving an existing LLM for a specific task or domain. You can improve an LLM by giving it a set of labeled examples for that task which it can then learn from. The examples can come from public datasets on the internet, or private datasets specific to your organization.
Together facilitates every step of the fine-tuning process. You can use our APIs for the following:
- Uploading your own datasets to our platform
- Starting a fine-tuning job that fine-tunes an existing LLM of your choice with your uploaded data
- Monitoring the progress of your fine-tuning job
- Hosting the resulting model on Together (or downloading it, so you can run it locally)
Together supports both LoRA fine-tuning and full fine-tuning. Get started fine-tuning a LLM with the following steps:
Choosing your model
The first step in fine-tuning is to choose which LLM you want to use as the starting point for your custom model.
All generative language models are trained to take some input text and then predict what text is most likely to follow it. While base models are trained on a wide variety of texts, making their predictions broad, instruct (or instruction-tuned) models are trained on text that's been structured as instruction-response pairs – hence their name. Each instruct model has its own input format; however, you only need to pass in the prompt
and completion
pairs or a list of message
objects — please refer to the data format details here.
If it's your first time fine-tuning LLMs, we recommend using an instruction-tuned model. meta-llama/Llama-3.2-3B-Instruct is great for simpler tasks, and the larger meta-llama/Llama-3.3-70B-Instruct-Reference is good for more complex datasets and domains.
You can find all available models on the Together API here.
Preparing your data
Once you've chosen your model you'll need to save your structured data as either a JSONL file or a Parquet (already tokenized) file.
Which file format should I use for data?
JSONL is simpler and will work for many cases, while Parquet stores pre-tokenized data, providing flexibility to specify custom attention mask and labels (loss masking). It also saves you time for each job you run by skipping the tokenization step. View our file format guide to learn more about working with each format.
By default, it's easier to use JSONL. However, there are a couple of things to keep in mind:
- For JSONL training data, we use a variation of sample packing that improves training efficiency by utilizing the maximum context length via packing multiple examples together. This technique changes the effective batch size, making it larger than the specified batch size, and reduces the total number of training steps.
If you'd like to disable packing during training, you can provide a tokenized dataset in a Parquet file. This example script for tokenizing a dataset demonstrates padding each example with a padding token. Note that the correspondingattention_mask
andlabels
should be set to 0 and -100, respectively, so that the model ignores the padding tokens during prediction and excludes them from the loss calculation. - If you want to specify custom
attention_mask
values or apply some tokenization customizations unique to your setup, you can use the Parquet format as well.
Loss masking
The Together Fine-tuning API trains a model using the same cross-entropy loss used during pre-training (in other words, by predicting the next token). However, in some cases, you may want to fine-tune a model to focus on predicting only a specific part of the prompt.
For example, if you're fine-tuning a model to answer a short question followed by a long context, the model doesn't need to learn to generate the question or the context, only the answer. Penalizing it for predictions over the context and question could lead to ineffective training for the answering task. In other words, you may want your model to use certain information without being trained to generate it.
Solution
- When using Conversationalor Instruction Data Formats, you can specify an argument
--train-on-inputs (bool or 'auto')
-- Whether to mask the user messages in conversational data or prompts in instruction data. Setting the parameter toyes
will mask out all the user messages. - When using Conversational format you can mask specific messages by assigning a weight to each one.
- Using pretokenized dataset(in a Parquet file). By providing a custom
labels
field for your examples in the tokenized dataset, you can mask out the loss calculation for specified tokens. Set the label for tokens you don’t want to include in the loss calculation to-100
(see here for why). Note that unlike padding tokens, you still set their correspondingattention_mask
to 1, so that the model can properly attend to these tokens during prediction.
Loss masking and truncation
By default, the Fine-tuning API truncates long sequences at the end for usual fine-tuning and at the beginning for the preference fine-tuning. When loss masking is applied, some input sequences may have all of their unmasked tokens truncated, which will cause the model not to learn anything.
File check
Once your data is in the correct structure and saved as either a .jsonl
or .parquet
file, use our CLI to verify that it's correct:
together files check "your-datafile.jsonl"
You'll see an object that looks like the following:
{
"is_check_passed": true,
"message": "Checks passed",
"found": true,
"file_size": 781041,
"utf8": true,
"line_type": true,
"text_field": true,
"key_value": true,
"min_samples": true,
"num_samples": 238,
"load_json": true,
"filetype": "jsonl"
}
If your data file is valid, you'll see is_check_passed: true
in the response.
You're now ready to upload your data to Together!
Uploading your data
To upload your data, use the CLI or our Python library (our TypeScript library currently doesn't support file uploads):
together files upload "your-datafile.jsonl"
import os
from together import Together
client = Together(api_key=os.environ.get("TOGETHER_API_KEY"))
resp = client.files.upload(file="your-datafile.jsonl")
print(resp.model_dump())
You'll see the following output once the upload finishes:
{
"id": "file-5e32a8e6-72b3-485d-ab76-71a73d9e1f5b",
"object": "file",
"created_at": 1713481731,
"type": null,
"purpose": "fine-tune",
"filename": "your-datafile.jsonl",
"bytes": 0,
"line_count": 0,
"processed": false,
"FileType": "jsonl"
}
You'll be using your file's ID (the string that begins with "file-") to start your fine-tuning job, so store it somewhere before moving on.
You're now ready to kick off your first fine-tuning job!
Starting a fine-tuning job
We support both LoRA and full finetuning; see how to start a fine-tuning job with either method below.
Hyperparameters
See the full list of hyperparameters and their definitions here.
LoRA fine-tuning
LoRA fine-tuning fine-tuning only a small subset of weights compared to full fine-tuning. We recommend using this method by default.
Call create
with your file ID as the training_file
to kick off a new fine-tuning job. Pass --lora
for LoRA fine-tuning:
together fine-tuning create \
--training-file "file-5e32a8e6-72b3-485d-ab76-71a73d9e1f5b" \
--model "meta-llama/Meta-Llama-3-8B" \
--wandb-api-key $WANDB_API_KEY \ # Optional
--lora
import os
from together import Together
client = Together(api_key=os.environ.get("TOGETHER_API_KEY"))
response = client.fine_tuning.create(
training_file = 'file-5e32a8e6-72b3-485d-ab76-71a73d9e1f5b',
model = 'meta-llama/Meta-Llama-3-8B',
lora = True,
n_epochs = 3,
n_checkpoints = 1,
batch_size = "max",
learning_rate = 1e-5,
suffix = 'my-demo-finetune',
wandb_api_key = '1a2b3c4d5e.......',
)
print(response)
--training-file
is the ID you received in the previous step
--model
one of the models from the Together API here
--wandb-api-key
a key to use for wandb
By default, we use the maximum possible batch size for the model (--batch-size max
) when you do not specify it.
You can also specify LoRA parameters --lora-r
, --lora-dropout
, --lora-alpha
, --lora-trainable-modules
to customize your job. See the full list of hyperparameters and their definitions here.
The response object will have all the details of your job, including its ID and a status
key that starts out as "pending":
{
"id": "ft-bb62e747-b8fc-49a3-985c-f32f7cc6bb04",
"training_file": "file-5e32a8e6-72b3-485d-ab76-71a73d9e1f5b",
"model": "meta-llama/Meta-Llama-3-8B",
"status": "pending"
}
Full fine-tuning
Call create
with your file ID as the training_file
to kick off a new fine-tuning job:
together fine-tuning create \
--training-file "file-5e32a8e6-72b3-485d-ab76-71a73d9e1f5b" \
--model "meta-llama/Meta-Llama-3-8B" \
--wandb-api-key $WANDB_API_KEY \ # Optional
import os
from together import Together
client = Together(api_key=os.environ.get("TOGETHER_API_KEY"))
response = client.fine_tuning.create(
training_file = 'file-5e32a8e6-72b3-485d-ab76-71a73d9e1f5b',
model = 'meta-llama/Meta-Llama-3-8B',
)
print(response)
import Together from 'together-ai';
const client = new Together({
apiKey: process.env['TOGETHER_API_KEY'],
});
const response = await client.fineTune.create({
model: 'meta-llama/Meta-Llama-3-8B',
training_file: 'file-5e32a8e6-72b3-485d-ab76-71a73d9e1f5b',
});
console.log(response);
See the full list of hyperparameters and their definitions here.
The response object will have all the details of your job, including its ID and a status
key that starts out as "pending":
{
"id": "ft-bb62e747-b8fc-49a3-985c-f32f7cc6bb04",
"training_file": "file-5e32a8e6-72b3-485d-ab76-71a73d9e1f5b",
"model": "meta-llama/Meta-Llama-3-8B",
"status": "pending"
}
Continue a fine-tuning job
You can continue training from a previous fine-tuning job by specifying the --from-checkpoint
parameter:
together fine-tuning create \
--training-file "file-5e32a8e6-72b3-485d-ab76-71a73d9e1f5b" \
--from-checkpoint "ft-bb62e747-b8fc-49a3-985c-f32f7cc6bb04" \
--wandb-api-key $WANDB_API_KEY # Optional
import os
from together import Together
client = Together(api_key=os.environ.get("TOGETHER_API_KEY"))
response = client.fine_tuning.create(
training_file = 'file-5e32a8e6-72b3-485d-ab76-71a73d9e1f5b',
from_checkpoint = 'ft-bb62e747-b8fc-49a3-985c-f32f7cc6bb04',
wandb_api_key = '1a2b3c4d5e.......',
)
print(response)
import Together from 'together-ai';
const client = new Together({
apiKey: process.env['TOGETHER_API_KEY'],
});
const response = await client.fineTune.create({
from_checkpoint: 'ft-bb62e747-b8fc-49a3-985c-f32f7cc6bb04',
training_file: 'file-5e32a8e6-72b3-485d-ab76-71a73d9e1f5b',
});
console.log(response);
You can specify a checkpoint by using:
- The output model name from the previous job
- Fine-tuning job ID
- A specific checkpoint step with the format
ft-...:{STEP_NUM}
, where{STEP_NUM}
is the step on which the checkpoint was created
- A specific checkpoint step with the format
To check all available checkpoints for the job, use:
together fine-tuning list-checkpoints {FT_JOB_ID}
Evaluation
What is a validation set?
A validation set is a held-out dataset to evaluate your model performance during training on unseen data. The validation set can be created from the same data source as the training dataset, or it can be a mix of multiple data sources. For example, you may include samples from various datasets to see if the model preserves its general capability while being fine-tuned for a specific task.
To use a validation set, provide --validation-file
and --n-evals
the number of evaluations (over the entire job). This needs to be set as a number above 0 in order for your validation set to be used. :
together fine-tuning create \
--training-file "file-5e32a8e6-72b3-485d-ab76-71a73d9e1f5b" \
--validation-file "file-44117187-5d76-4915-9b4c-bdd73f33498e" \
--n-evals 10 \
--model "meta-llama/Meta-Llama-3-8B" \
--wandb-api-key $WANDB_API_KEY \ # Optional
import os
from together import Together
client = Together(api_key=os.environ.get("TOGETHER_API_KEY"))
response = client.fine_tuning.create(
training_file = 'file-5e32a8e6-72b3-485d-ab76-71a73d9e1f5b',
validation_file = 'file-44117187-5d76-4915-9b4c-bdd73f33498e',
n_evals = 10,
model = 'meta-llama/Meta-Llama-3-8B',
)
print(response)
See the full list of hyperparameters and their definitions here.
What is a validation set?
A validation set is a held-out dataset to evaluate your model performance during training on unseen data. The validation set can be created from the same data source as the training dataset, or it can be a mix of multiple data sources. For example, you may include samples from various datasets to see if the model preserves its general capability while being fine-tuned for a specific task.
How often is the evaluation run on the validation set?
At a set number of training steps, defined by your input n_evals
, the most up-to-date model weights will be evaluated with a forward pass on your validation set, and the evaluation loss will be recorded in your job event log. If you provide a W&B API key, you will also be able to see the losses in the W&B page. Therefore, the presence of the validation set will not influence the model's training quality.
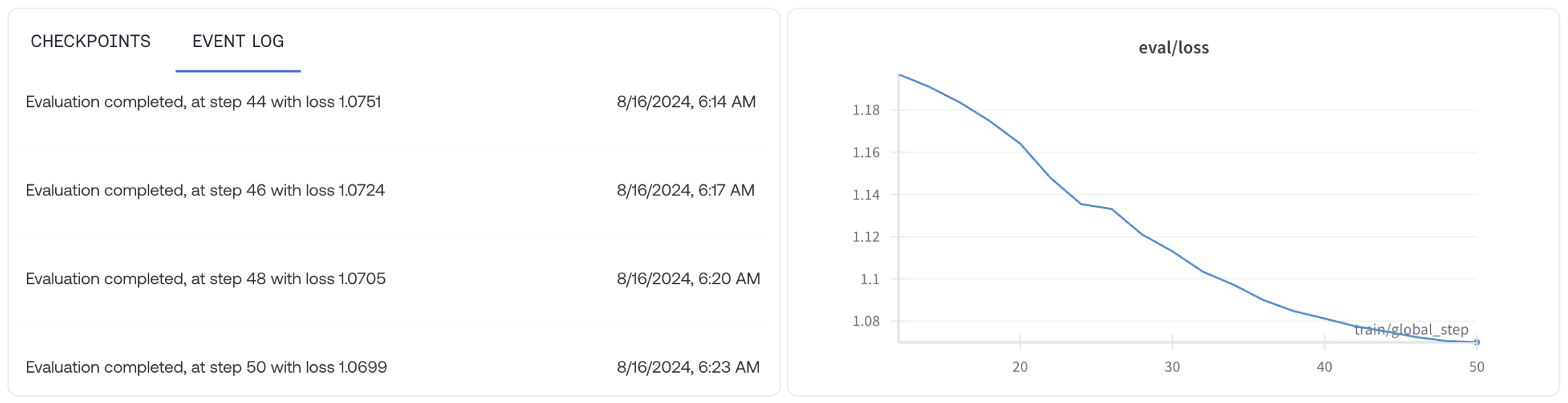
So, when exactly is the evaluation performed? To ensure that the final weights are evaluated on the validation set, the counting for evaluation steps may start after a few training steps. In the example below, the evaluation is performed every 7 training steps with the step 9 being the first evaluation and the final step 30 being the last evaluation.
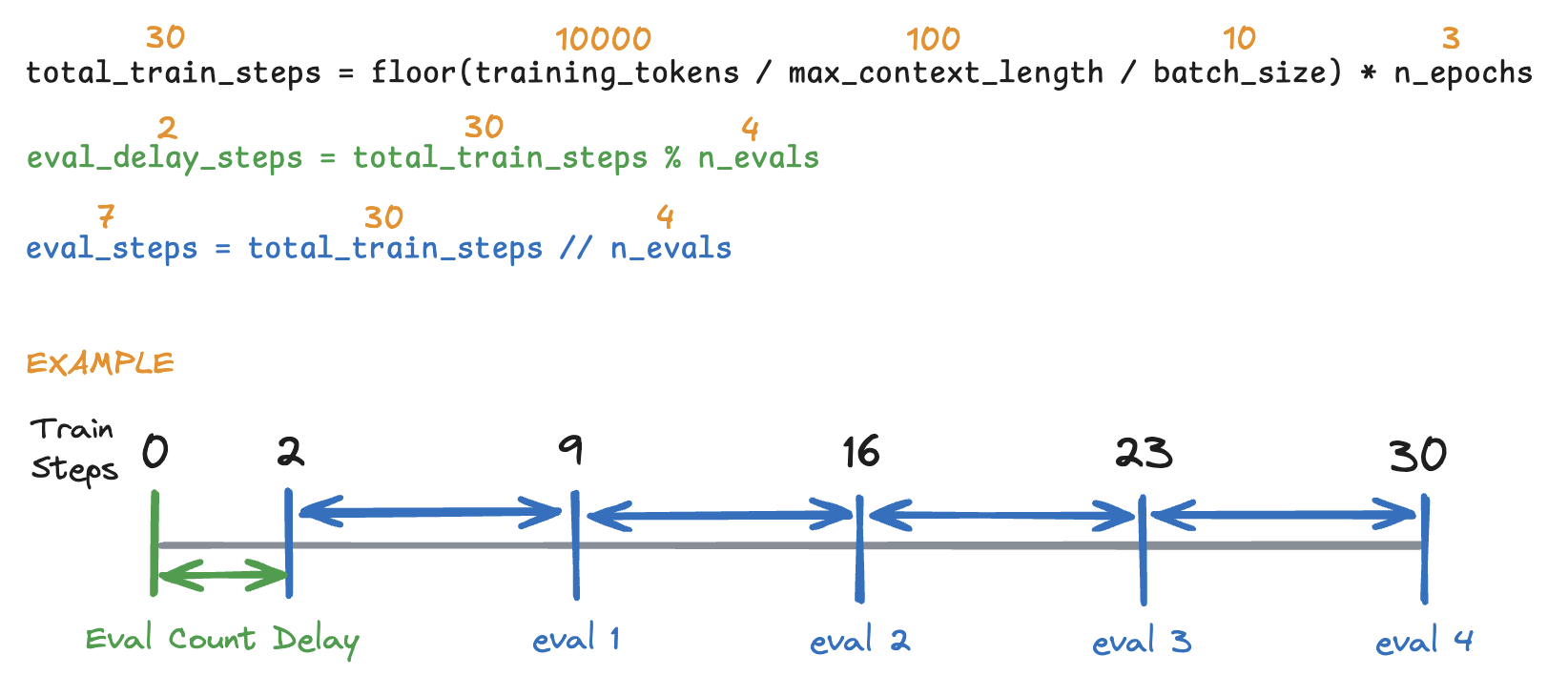
Why should I provide a validation set?
Using a validation set during training provides multiple benefits such as hyperparameter tuning and examining model performance on unseen data, helping you identify if the model is overfitting.
Note that the evaluation cost will be added to your final cost based on the size of your validation set and the number of evaluations. To get more details, see the pricing section.
Training and Validation Split
You can split a JSONL file for training and validation by running the following example script.
split_ratio=0.9 // Specify the split ratio for your training set.
total_lines=$(wc -l < "your-datafile.jsonl")
split_lines=$((total_lines * split_ratio))
head -n $split_lines "your-datafile.jsonl" > "your-datafile-train.jsonl"
tail -n +$((split_lines + 1)) "your-datafile.jsonl" > "your-datafile-validation.jsonl"
Monitoring a fine-tuning job's progress
After you started your job, visit your jobs dashboard. You should see your new job!
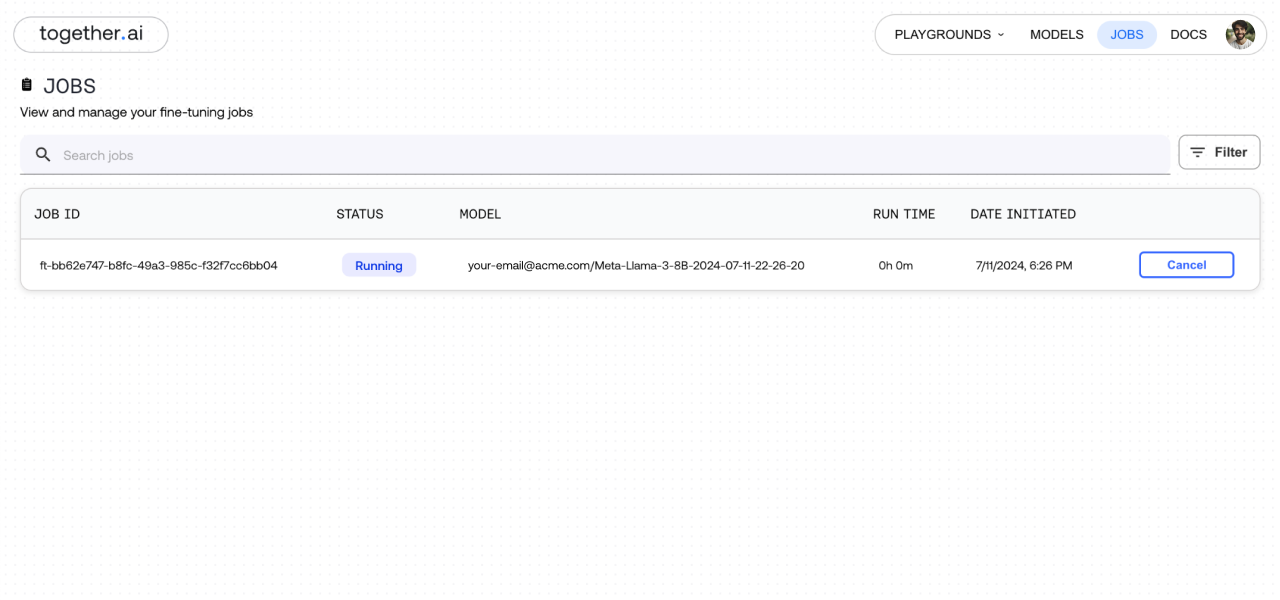
You can also pass your Job ID to retrieve
to get the latest details about your job directly from your code:
together fine-tuning retrieve "ft-bb62e747-b8fc-49a3-985c-f32f7cc6bb04"
import os
from together import Together
client = Together(api_key=os.environ.get("TOGETHER_API_KEY"))
response = client.fine_tuning.retrieve('ft-bb62e747-b8fc-49a3-985c-f32f7cc6bb04')
print(response.status) # STATUS_UPLOADING
import Together from 'together-ai';
const client = new Together({
apiKey: process.env['TOGETHER_API_KEY'],
});
const response = await client.fineTune.retrieve(
'ft-bb62e747-b8fc-49a3-985c-f32f7cc6bb04'
);
console.log(response.status); // uploading
Your fine-tuning job will go through several phases, including Pending, Queued, Running, Uploading, and Completed. You can check the current status at any time by visiting your jobs dashboard or using the retrieve
command from above. If your job is in a pending state for too long, please reach out to [email protected].
You can also monitor the fine-tuning job on the Weights & Biases platform, shown below, if you provided your API key when submitting the fine-tuning job as instructed above. Fine-tuning jobs will appear in the "together"
project if another location was not specified.
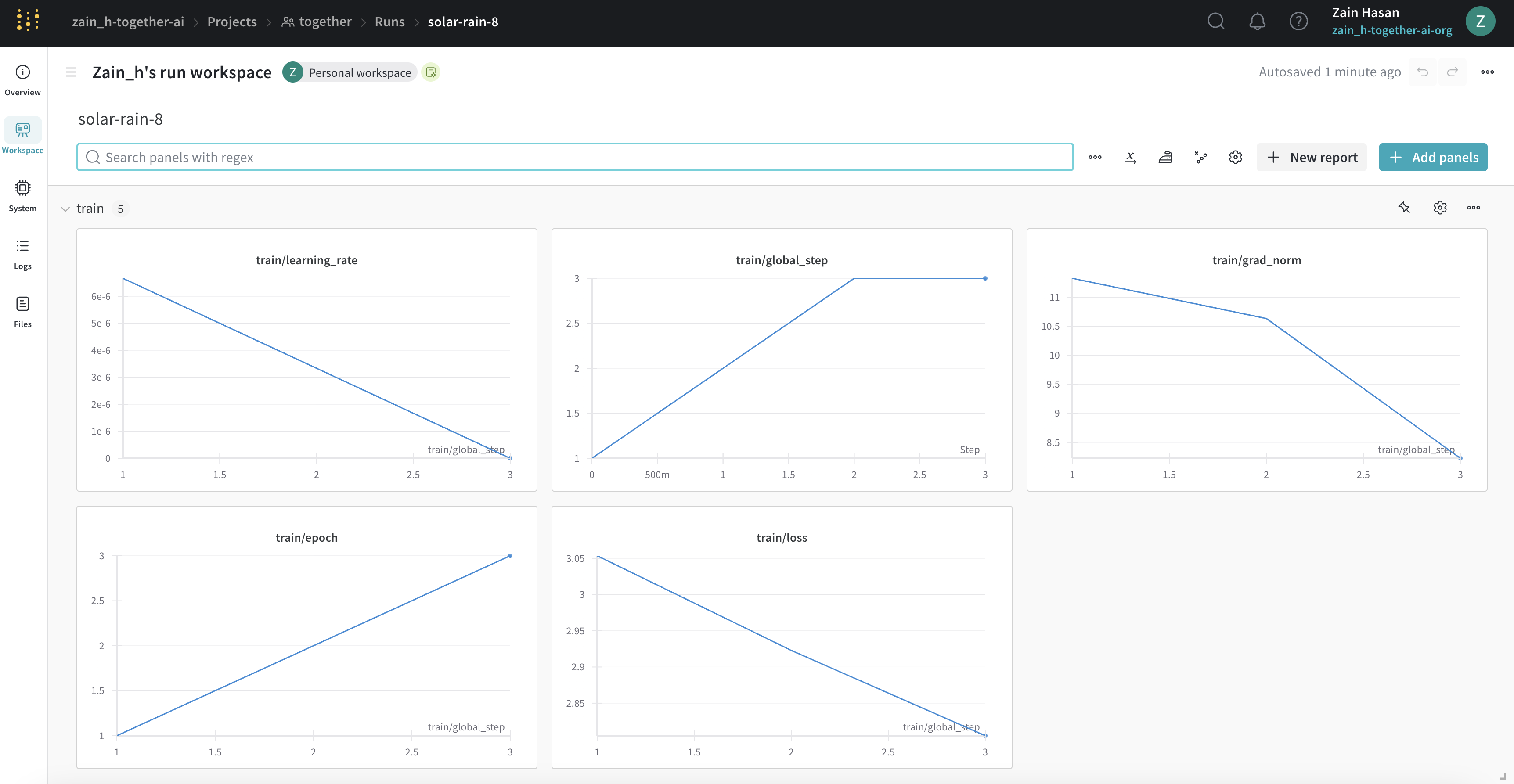
When the status says Completed, your job is all done! You've just fine-tuned your first model with the Together API, and now you're ready to deploy it.
Deploying your fine-tuned model
Once your fine-tune job completes, you should see your new model in your models dashboard.

To use your model, you can either host it on Together AI for an hourly usage fee, or download your model and run it locally. Currently, there is no difference between hosting LoRA fine-tuned models and hosting full fine-tuned models.
Read more about the deployment of a fine-tuned model here.
Pricing
Read more about pricing here.
Updated about 1 month ago